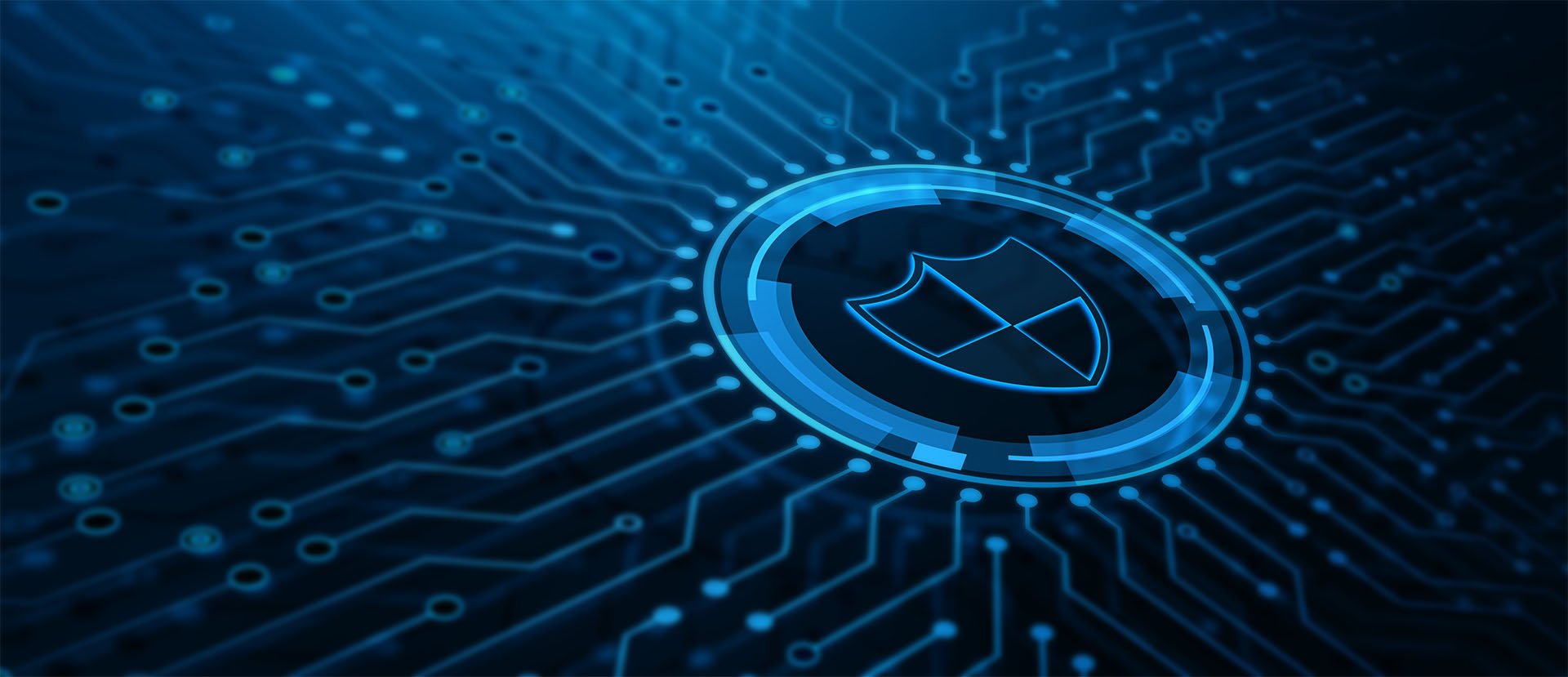
Giacomo Iadarola, Fabio Martinelli, Francesco, Antonella Santone
Computer & Security, 2021
DOI: https://doi.org/10.1016/j.cose.2021.102198
Mobile devices are pervading the everyday activities of our life. Each day we store a plethora of sensitive and private information in smart devices such as smartphones or tablets, which are typically equipped with an always-on internet connection. This information is of interest for malicious writers that are developing more and more aggressive harmful code for stealing sensitive and private information from mobile devices. Considering the weaknesses exhibited from current antimalware signature-based detection, in this paper, we propose a method relying on application representation in terms of images used to input an explainable deep learning model designed by authors for Android malware detection and family identification. Moreover, we show how the explainability can be considered by the analyst to assess different models. Experimental results demonstrated the effectiveness of the proposed method, obtaining an average accuracy ranging from 0.96 to 0.97; we evaluated 8446 Android samples belonging to six different malware families and one more family for trusted samples, by providing also interpretability about the predictions performed by the model.
Cyberwatching.eu has received funding from the European Union’s Horizon 2020 research and innovation programme under grant agreement No 740129. The content of this website does not represent the opinion of the European Commission, and the European Commission is not responsible for any use that might be made of such content. Privacy Policy | Disclaimer / Terms and Conditions of Use