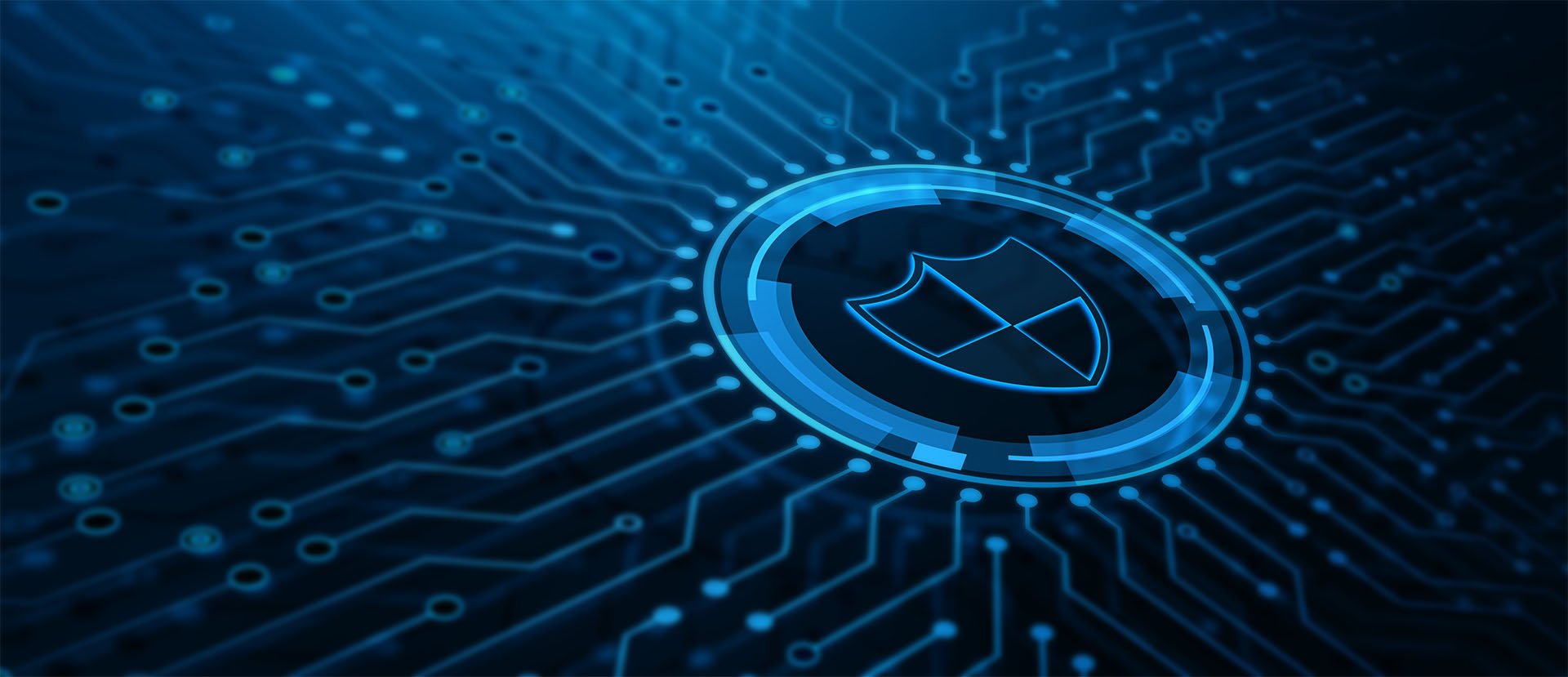
Fabio Martinelli, Francesco Mercaldo, Domenico Raucci, Antonella Santone
Workshops of the International Conference on Advanced Information Networking and Applications
WAINA 2020: Web, Artificial Intelligence and Network Applications pp 959-969
https://doi.org/10.1007/978-3-030-44038-1_87
Data mining techniques were employed to automatise decision-making processes in several domains. In the banking context, the introduction of IFRS 9 on Financial Instruments has impacted not only on the area of accounting and financial reporting, but also on banks’ credit risk measurement and management processes, promoting effective and efficient data mining applications. In detail, banking management can benefit from these techniques by extracting knowledge from data to support more advanced models, in particular for the assessment of credits deriving from lending activity, in accordance with the Expected Loss Approach provided by the new standard. In this study, we exploit data mining techniques to measure the probability of default of credits with specific features at the reporting date. We consider supervised machine learning to build predictive models and association rules to infer a set of rules by a real-world data-set, reaching interesting results in terms of accuracy.
Cyberwatching.eu has received funding from the European Union’s Horizon 2020 research and innovation programme under grant agreement No 740129. The content of this website does not represent the opinion of the European Commission, and the European Commission is not responsible for any use that might be made of such content. Privacy Policy | Disclaimer / Terms and Conditions of Use