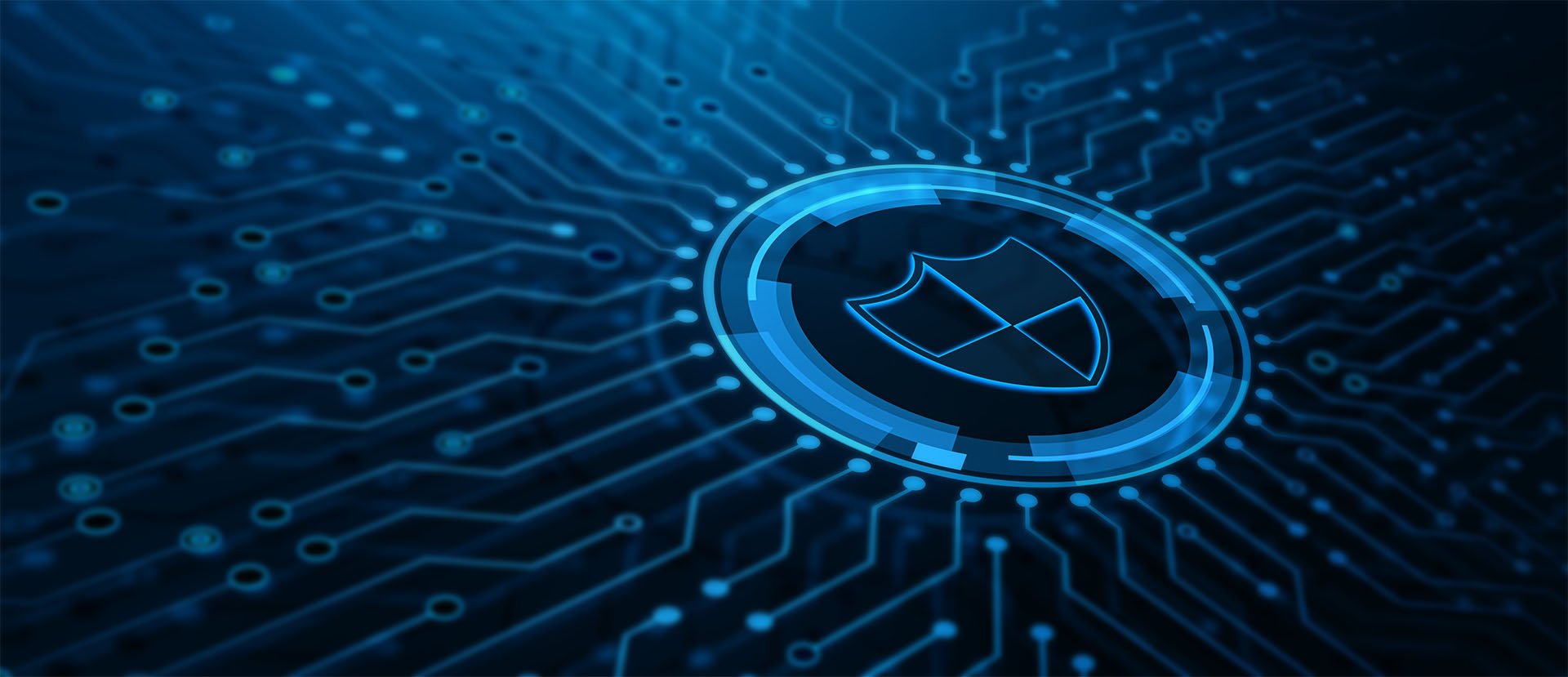
Giacomo Iadarola, Fabio Martinelli, Francesco Mercaldo, Antonella Santone
IEEE International Symposium on Software Reliability Engineering Workshops (ISSREW), Coimbra, Portugal, 2020, pp. 255-260
https://doi.org/10.1109/ISSREW51248.2020.00082
Artificial intelligence techniques are nowadays widespread to perform a great number of classification tasks. One of the biggest controversies regarding the adoption of these techniques is related to their use as a “black box” i.e., the security analyst must trust the prediction without the possibility to understand the reason why the classifier made a certain choice. In this paper we propose a malicious family detector based on deep learning, providing a mechanism aimed to assess the prediction reliability. The proposed method obtains an accuracy of 0.98 in Android family identification. Moreover, we show how the proposed method can assist the security analyst to interpret the output classification and verify the prediction reliability by exploiting activation maps.
Cyberwatching.eu has received funding from the European Union’s Horizon 2020 research and innovation programme under grant agreement No 740129. The content of this website does not represent the opinion of the European Commission, and the European Commission is not responsible for any use that might be made of such content. Privacy Policy | Disclaimer / Terms and Conditions of Use